Try Default
See how revenue teams automate revenue operations with Default.
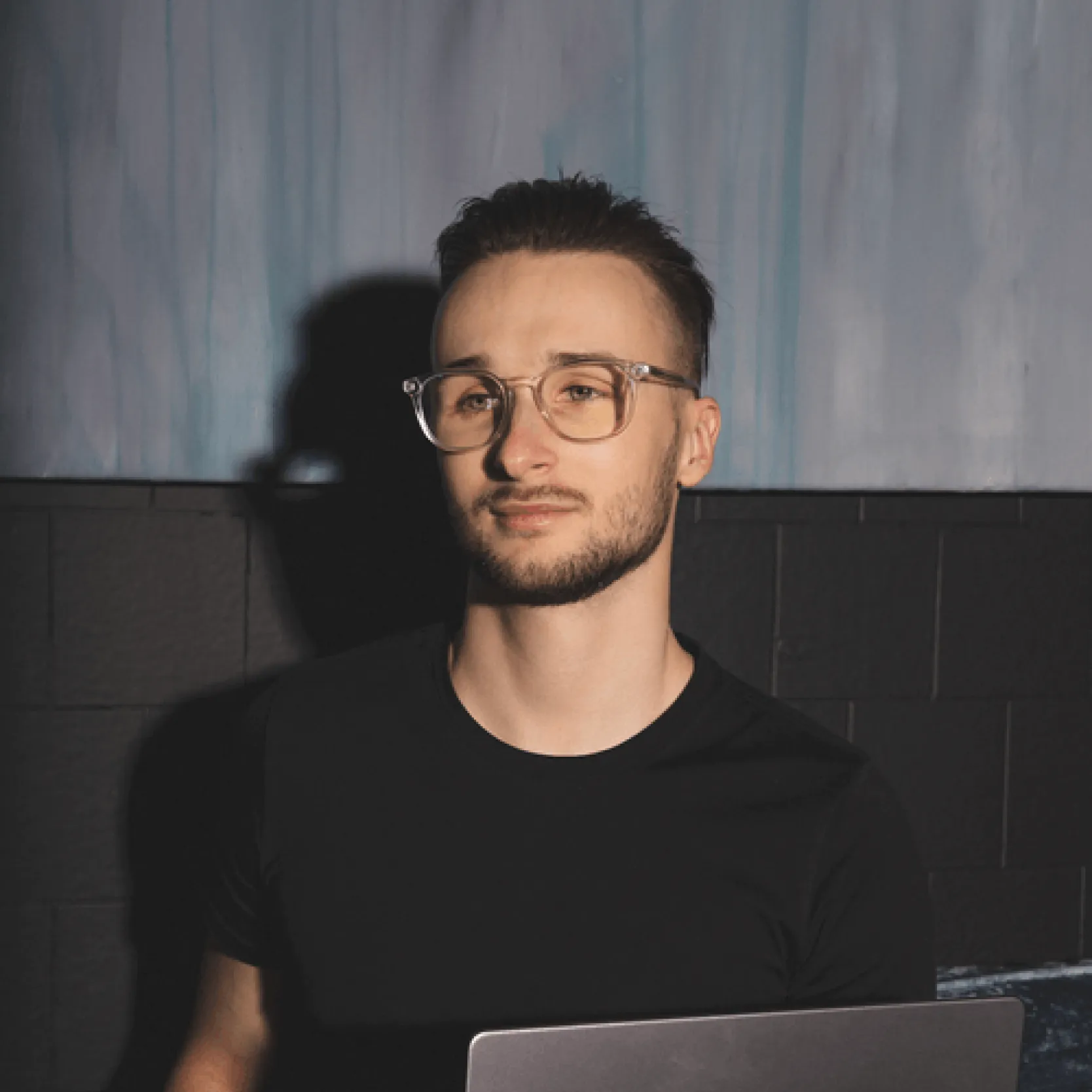
Key Takeaways
Right now, sales organizations are taking one of two approaches to AI. Some are adopting the AI SDR approach: using AI to replace front-line human sales reps. Others (like Default) are focusing on the back-end: using AI to automate monotonous sales tasks. AI lead scoring is one example of this latter category.
If AI lead scoring isn’t part of your RevOps tech stack, it’s time to make it a priority. This article will define AI lead scoring, walk through its benefits, and show you how it works.
What is AI lead scoring?
AI lead scoring is the process of using prospect and customer data to prioritize leads based on their likelihood to convert into a customer. Lead scoring is a critical part of the inbound lead qualification process that helps automatically determine whether to send a newly created lead to the sales team.
There are multiple ways to use AI in lead scoring, and different RevOps tech platforms will deploy them in different ways:
- Aggregate & summarize hundreds of data points per contact record and, based on a predetermined scoring system, determine whether that lead is likely to close
- Analyze thousands (sometimes millions) of data points across your existing customers to develop a model that predicts whether a lead is likely to close
- Generate new leads by applying your prediction model to third-party contact lists (this use case is generally only available with enterprise-level platforms)
Regardless of which approach your lead qualification software adopts, AI lead scoring can accelerate the manual processes involved in prioritizing leads. That way, your sales teams don’t waste time with unqualified meetings.
Default aggregates first- and third-party data to provide built-in lead qualification. The platform also integrates with a variety of scoring models. See how we score leads through our built-in lead enrichment software here.
Manual vs. AI lead scoring: Our comparison
Lead scoring is nothing new in the digital marketing world. Organizations have been doing manual lead scoring ever since inbound marketing became common practice, if not before.
What’s new in recent years, however, is the use of AI in accelerating the lead scoring process through one of the three methods listed above. Let’s take a look at how each process works and their advantages and disadvantages.
Advantages of manual lead scoring
- Sales reps can pull both quantitative and qualitative data to provide a full picture of a lead’s attributes, including insight into how to follow up with leads based on where they sit in the buying journey
- Improved ability to contextualize data gathered from enrichment
- Easier to translate insights from lead scoring into conversations with leads, as it’s the same person performing both tasks
- Greater control and customization over the scoring process
- Easier to account for outliers and edge cases that could turn into fruitful opportunities
Disadvantages of manual lead scoring
- Difficult to scale, as it’s time-consuming and resource-intensive
- Opportunity cost of reps spending time on lead scoring rather than prospecting and closing new business
- Subject to human error and bias, especially if you hand it off to a junior sales rep with limited experience or industry knowledge
- Slows down the qualification and routing process, which decreases your speed-to-lead
Advantages of AI lead scoring
- Faster than manual scoring, as leads can be enriched, scored, qualified, and routed within minutes
- Near-infinite scalability, as you’re not relying on additional personnel to handle increased volumes of inbound leads
- Applies a single scoring model consistently, removing human error and bias from the qualification process (although human error and bias can be baked into the model)
- Predictive models that are regularly retrained can incorporate changing customer data into future iterations of the model, thus remaining dynamic to changing customer dynamics
Disadvantages of AI lead scoring
- Can often miss important qualitative data, nuances, market trends, and other contextual information that only human intuition can pick up
- Errors and biases baked into the scoring model are applied across the entire sales funnel at all times
- Predictive models can be prone to confirmation bias: you only attract customers who fit your current customer profile, which hinders your ability to expand your customer base
- Often struggles with outliers (unless rules for handling outliers are built into the model)
7 benefits of AI lead scoring
In our experience, the best RevOps software (including Default) has some kind of AI lead scoring features built into the platform. Given that “AI” is a bit of a buzzword right now, let’s walk through how it can actually accelerate revenue and add value to your business.
Find out in our guide how Hubspot lead scoring works.
1. Faster speed-to-lead
AI lead scoring is fast. In fact, we’d be hard-pressed to find anyone who can manually score a lead faster than an AI model can. In fact, our proprietary study of over 88K inbound leads shows that AI-powered features reduce the time it takes to service an inbound lead by 31%.

The question regarding the value of AI lead scoring is about accuracy, not speed. And that’s a reasonable concern. Sending leads to sales that never close will actually slow revenue, rather than accelerate it.
One way to avoid this problem is to measure qualification rates against second meeting or opportunity creation rates. If you have a bunch of “qualified” leads that don’t convert into sales, that’s a sign you need to reconfigure your model.
But assuming your model is accurate, it can save hours of your sales team’s time per week and get meetings on the calendar faster. The downstream effect, of course, being accelerated pipelines and revenue.
2. Resource efficiency
Many sales teams are using AI to replace their human sales reps. The rise of AI SDR tools is one example of this phenomenon, but we’re bound to see others in the future.
At Default, we’re not convinced that’s the best use of AI. Rather than try and replace SDRs or AEs, AI should enable you to do more with the team you currently have.
For example, an SDR can use AI lead scoring to automatically route those “slam dunk” leads that have come in. With all that time they’ve saved, these SDRs could handle the leads that are “on the fence” or even outliers, determining where to route them and what kind of value they might offer.
Additionally, AI lead scoring can scale up rote tasks like lead-to-account matching, enabling you save significantly on time and resources invested into CRM and database management.
3. Scalability
There’s no hard upper limit on how many leads AI lead scoring can handle. Those limits are typically set by your software provider and pricing plan, which in turn are set by their database administrators and IT providers.
But as with any cloud platform, there’s no limit to how high you can go if you have the resources to spend. And nine times out of ten, you can scale an AI lead scoring solution faster and cheaper than hiring more sales reps.
This means you can start with an AI lead scoring solution that works for your early days as a business, then scale that up as your organization grows. Keeping the same solution in place over the life of your business can help you avoid costly and annoying future tech stack migrations.
4. Scoring consistency
AI lead scoring models are consistent. They apply the same standards to every lead that comes to them. So you’re not going to miss opportunities due to human error and bias.
But you could potentially miss out on leads due to model inflexibility. Let’s say you have an ICP that includes companies with $10M in revenue or more. You may get an inbound lead with $5M in revenue, but they’re backed by a reputable VC firm and have solid financials.
An AI lead scoring model that isn’t built to handle outliers would miss that opportunity. So while consistency is important, it’s also important to build flexibility into your lead scoring model and sales workflow software.
5. Improved revenue team alignment
Marketing and sales teams are infamous for constantly being at odds. There are a number of reasons for this, but often it comes down to different definitions of success: Marketing wants to ship as many MQLs as possible, while Sales only wants to spend time with leads that require the least effort to close.
This often plays out in the lead qualification process. The definitions of a marketing qualified lead (MQL) vs. sales qualified lead (SQL) don’t always align, resulting in confusion and conflict on both sides.
AI lead scoring enables a layer of objectivity that can help to improve alignment and mitigate disagreements. For this to work, however, both teams need to be bought into the AI model before it’s implemented. It can’t be a “marketing solution” or “sales solution.” It needs to be a revenue solution.
6. Improved forecasting accuracy
AI lead scoring doesn’t just use or interpret data: it can often create new data to aid in your organizational decision making. The lead scores generated through the process can correlate with other business metrics—ARR, MRR, Weighted Pipeline Value, LTV, etc.—to predict where those metrics will land six, nine, or 12 months into the future.
This can be especially helpful for organizations engaging in enterprise SaaS sales and, thus, longer sales cycles. Let’s say that you have 100 MQLs come in during Q1. If you have a six-month sales cycle, you may not know whether those leads will convert into customers until the end of Q3. But you can’t afford to wait that long—you need to know the value of those leads so you can plan your Q2 motions accordingly.
Most organizations will apply a standard MQL → Opportunity conversion rate to pipeline value to forecast the revenue coming from those leads. However, this approach is like painting with a broad brush. Even leads that are “qualified” vary in terms of their:
- Enthusiasm, education, and overall awareness of the product
- Stage in the buying journey (i.e. “just gathering quotes” vs. “ready to buy now”)
- Authority within the buying organization
- Potential LTV of the account (e.g. a $10M vs. $100M company)
Lead scoring can offer greater insight into these distinctions. Here are some example correlations that are relevant to predicting pipeline value and speed:
- Leads scoring 100 and above close within 90 days, while leads scoring less than 100 close no sooner than six months
- Leads scoring 115 and above have churn rate of 30%, while leads scoring less than 100 have a churn rate of 50%
- Leads scoring 90 and above have an ACV of $50,000+, while leads scoring less than 90 have an ACV of $10,000
Obviously, the difference in those metrics can significantly impact your pipeline value. Using lead scoring to parse out those nuances, then, can provide greater accuracy and insight into where you currently stand.
7. Iterative improvements
We hinted at this above, but it’s worth mentioning in detail here. Depending on which model you use, AI lead scoring can be subject to iterative improvements. As it handles more test cases and receives feedback from your customer database (e.g. close rates, ACVs, churn rates), the model can incorporate that data and work to more accurately score leads.
For aggregation models, this can also help to adjust your expectations regarding who your ICP actually is, versus who you think they are when you first start planning your marketing strategy. If you find that your target customer is a smaller-deal, more transactional buy vs. consultative enterprise sales, then you’ll want to adjust your model to score based on who’s actually buying.
For predictive models, it’s important that you’re continually feeding new data into your model as you win and lose customers. Without that key insight, you’ll end up scoring leads on old data, which will kill your model’s accuracy.
How AI lead scoring works
As we mentioned above, AI lead scoring works in three ways: aggregation, prediction, and generation. From a technical perspective, there are a number of mechanisms that enable it to accomplish any and all of these ends.
Large datasets
For AI lead scoring to work effectively, you need to have access to large datasets to analyze it. Not only do you need hundreds of contacts in your database, but also hundreds of datapoints (typically captured through enrichment) on those leads.
A typical AI lead scoring model will deploy the following data operations:
- Data collection. This is where the model will collect data from across your RevOps tech stack. The more data it collects, the more accurate the model will be.
- Data analysis. The next step is to identify patterns and correlations that aid in predicting positive outcomes.
- Continuous learning. As more data becomes available, the AI lead scoring model will refine itself and become more accurate.
If you use a third-party lead scoring model, you can get the benefit of an “out-of-the-box” solution that’s been trained on more data than you have access to. Regardless of which approach you take, however, the basic rule of thumb is: the more (accurate, relevant) data you feed it, the better your scoring will be.
Advanced AI/ML algorithms
Once you have those large datasets in place, AI lead scoring will deploy advanced artificial intelligence (AI) and machine learning (ML) algorithms to analyze those datasets. There are a number of models you can deploy, depending on the outcomes you want to achieve.
Purchase intent lead scoring
This lead model prioritizes intent data over ICP data. The goal is to identify those prospects who are ready to buy, regardless of how well they fit or align with your current customer base. This AI lead scoring model can be particularly helpful for early-stage organizations who are still figuring out their ideal customer profile, and mature organizations looking to expand into new verticals.
Ideal customer profile (ICP) lead scoring
For organizations that have a clear value proposition for a particular buyer persona and want to scale that particular customer base, an ICP lead scoring model can be helpful. This model weighs demographic and firmographic data over intent data.
This approach can also be helpful for enterprise or account-based selling (ABS) approaches, as you’re not necessarily interested in the short-term interest but in building the relationship over time.
Engagement lead scoring
This model looks primarily at engagement with your content, website, and branded channels, prioritizing leads that have a higher affinity with your brand. This can be helpful in highly competitive verticals, where brand equity can be the deciding factor between two similar products.
Negative lead scoring
Saying “no” can be as informative as saying “yes.” A negative lead scoring model looks at your churn and closed-lost data and identifies leads that are unlikely to convert or have a high LTV. This approach can be combined with any or all of the three models listed above, helping to surface unqualified leads.
There are many benefits to engaging with unqualified leads. Industry experts, potential funding partners, non-competitive peers, and other valuable relationships can come through unqualified leads. If you have specific workflows for routing and managing these contacts, a negative lead scoring model can help you scale up those efforts.
Real-time scoring
AI lead scoring works 24/7. No matter when your leads come in, your model can pull in enrichment data, score and qualify the lead, then—and this is the most important part—route and book a calendar meeting in real time. This allows for fast speed-to-lead, increasing your odds of a qualified meeting and, by extension, opportunity.
AI lead scoring FAQs
What is an example of a lead scoring system?
Here’s an example of a lead scoring system. If you use an engagement model, your AI lead scoring model will look at email open rates, click rates, social media likes and comments, website visits, number of pageviews, etc. Then it will look at the engagement rates of your customers, then score leads based on how closely their engagement aligns with these new leads. The closer the alignment, the higher the score.
What is Einstein lead scoring?
Einstein is Salesforce’s AI lead scoring tool. It’s only available with the Lightning Experience plan, or as an add-on for their standard Salesforce Editions (Essentials, Starter, Pro Suite, Professional, Enterprise, Unlimited).
Automate your lead scoring with Default
If you’re ready to start automating your inbound sales, AI lead scoring is an absolute must-have feature. Without automated lead scoring, your qualification, routing, scheduling, and nurture processes will be delayed—hurting your chances of pipelining and closing those deals.
At the same time, AI lead scoring won’t work unless it’s integrated into an automated process to service those qualified leads. So you need both sides of the equation.
Default is the only all-in-one sales workflow software that handles every aspect of the customer journey: conversion, enrichment, lead-to-account matching, scoring, qualification, routing, scheduling, follow-up, and nurture.
Conclusion
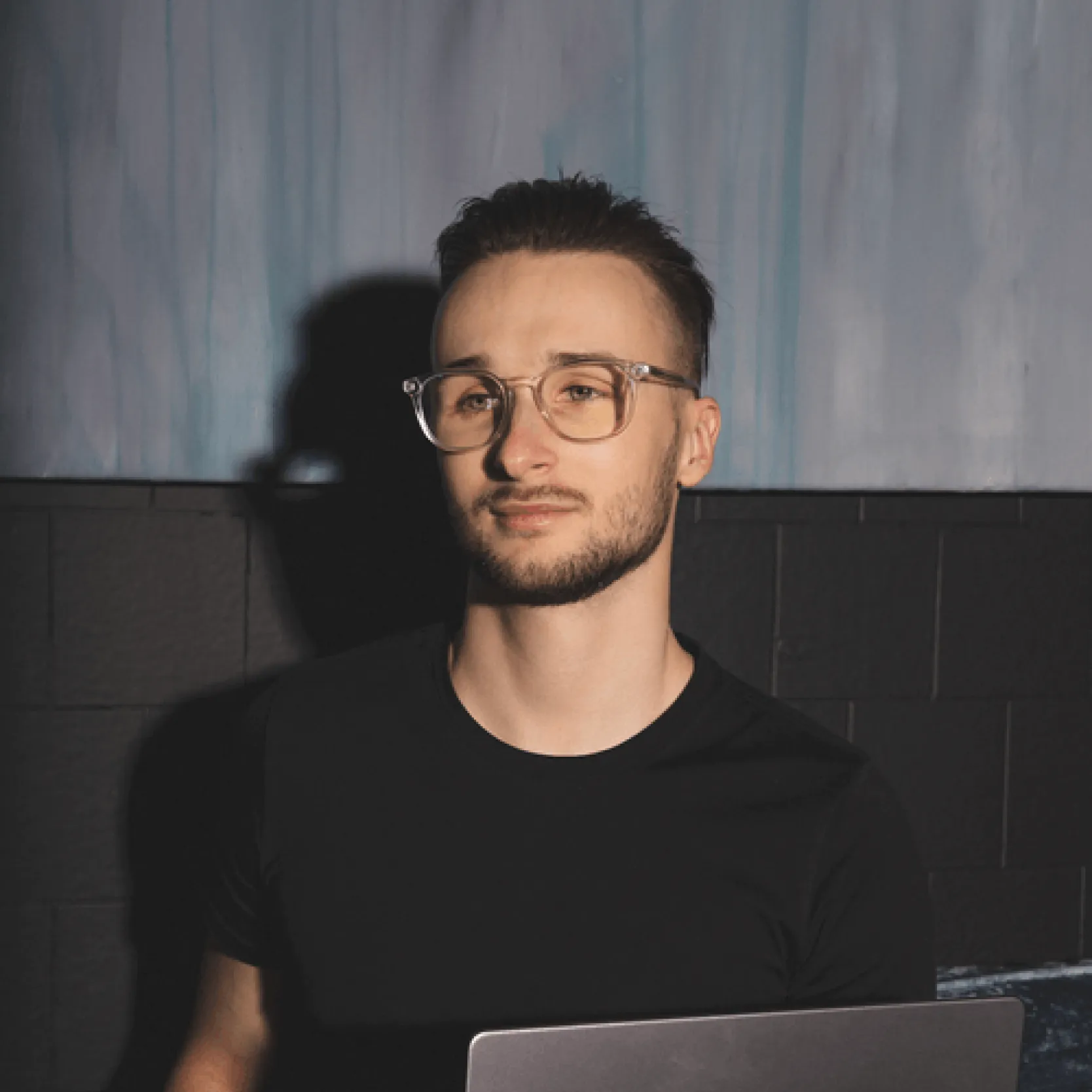
Former pro Olympic athlete turned growth marketer! Previously worked at Chili Piper and co-founded my own company before joining Default two years ago.
Accelerate your growth with Default.
Revamp inbound with easier routing, actionable intent, and faster scheduling